Ideally, policy and program design is a straightforward process: a decision-maker faces a problem, turns to peer-reviewed literature, and selects interventions shown to work. In reality, that’s rarely how things unfold. The popularity of “evidence-based medicine” and other “evidence-based” topics highlights our desire for empirical approaches — but would the world actually improve if those in power consistently took social science 1 evidence seriously? It brings me no joy to tell you that, at present, I think the answer is usually “no.”
Everyone likes the idea of evidence-based policy, but it’s hard to realize it when our most reputable social science journals are still publishing poor quality research.
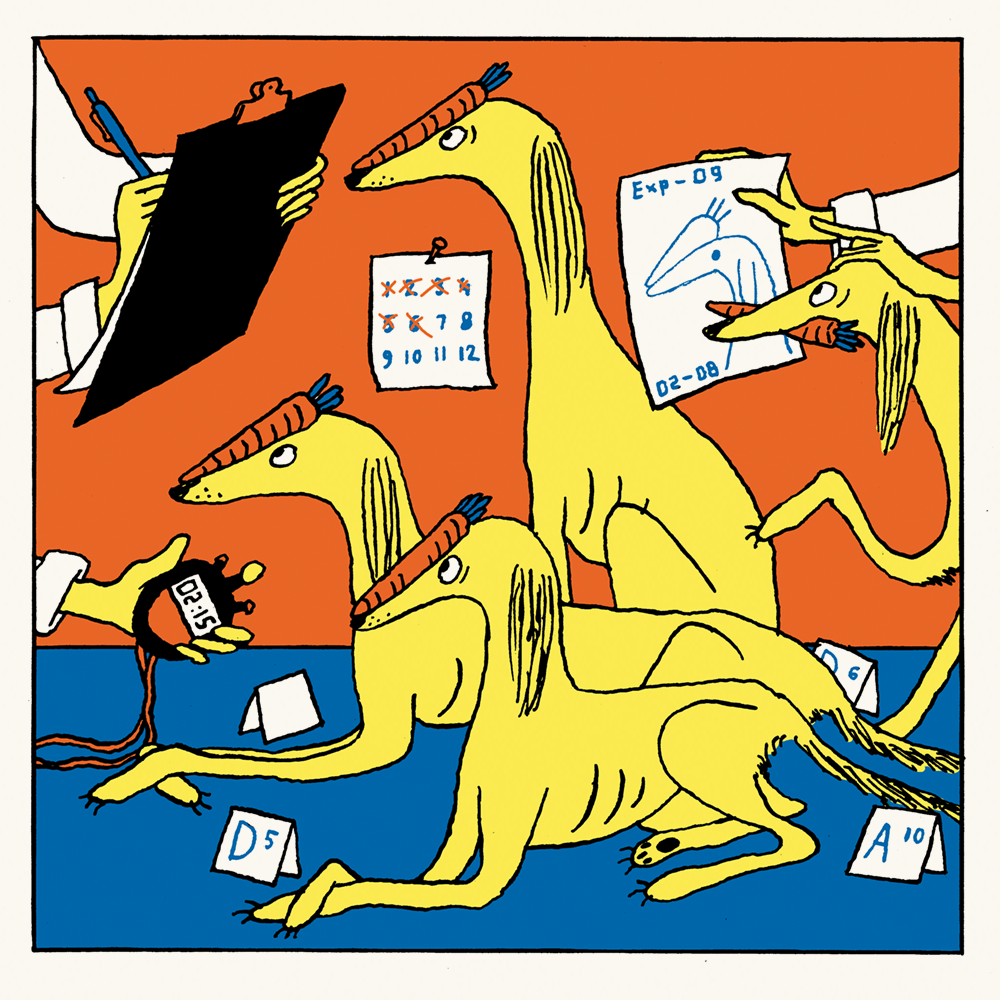
Given the current state of evidence production in the social sciences, I believe that many — perhaps most — attempts to use social scientific evidence to inform policy will not lead to better outcomes. This is not because of politics or the challenges of scaling small programs. The problem is more immediate. Much of social science research is of poor quality, and sorting the trustworthy work from bad work is difficult, costly, and time-consuming.
But it is necessary. If you were to randomly select an empirical paper published in the past decade — including any studies from the top journals in political science or economics — there is a high chance that its findings may be inaccurate. And not just off by a little: possibly two times as large, or even incorrectly signed. As an academic, this bothers me. I think it should bother you, too. So let me explain why this happens.
Does the code even run?
The most basic test of the reliability of social scientific research is making sure that the code used in an analysis 1) runs and 2) actually produces the given results. How often is this (low) standard met? A 2018 study looked across social science disciplines and found sobering results. First, even having code to replicate isn’t always a requirement. Few journals in psychology or sociology required authors to submit replication packages. Economics and political science journals tended to have stricter requirements, but still, these were uneven.
When the authors excluded their analysis to studies in highly ranked economics journals, only 29 out of the 203, or 14%, provided code that ran without errors and reproduced the final results from the supplied raw data. 2 This isn’t on its own damning; authors often share cleaned data instead of raw data. When working from this cleaned data instead of the raw data, the results were reproducible in 37% of papers. This is better, but it requires one to trust both that the data cleaning process was done correctly, and in a manner that would not substantively affect the results.
Of course, academia has been aware of the replication crisis since at least the early 2010s, and practices and processes seem to have improved since 2018. A 2024 study led by Abel Brodeur found that 85% of papers in top economics and political science contained code that ran properly (with minor modifications) and produced the results stated in the paper. Much of this improvement is a result of top journals implementing policies to check that the code runs. However, while these policies have become more common in top journals (77% of the papers in this study were published in journals with such policies), they remain rare most other places. And of course, merely running and producing the results in the paper is the lowest possible bar to clear — and 15% of papers in our best journals still can’t clear it.
Code that runs can still contain errors. For example, a hypothetical bug that duplicates data will not stop code from executing, but will result in erroneous estimates or standard errors. Brodeur’s team found this sort of flaw in about a quarter of the studies they looked at. Some examples of errors included “a very large number of duplicated observations, failing to fully interact a difference-in-differences regression specification, and miscoding the treatment variable for a large number of (or all) observations.” 3
I’m one of many co-authors on this meta-study. When I’ve discussed it with other academics, I’ve been surprised to find almost everyone interprets these results as good news. Most of them assumed the situation was even worse.
So already — with only our first and lowest hurdle — our policy-maker cannot have well-founded trust in most articles published before roughly 2016. But even since then, and even in our top journals, there’s still a 1 in 4 chance that the presented results are impacted by material errors.
Fragile results
The final result of a paper is the always the product of numerous analytical choices. For example, authors often consider excluding some observations, changing control variables, transforming different variables by cutting them into bins, or subsetting the data in numerous ways.
Thus, once we’ve determined that code works, the next step is to see if the results hold under a different set of analytical decisions. Sometimes, authors intentionally fish around to find the method that produces the best-looking numbers. More often, the fishing is less clearly fraudulent, though it’s just as damaging for the scientific record. Studies suggest that researchers who engage in this kind of data torturing do so unconsciously, apparently under the assumption that they are uncovering the truth. And it is more common still for researchers, over the period of years spent working on a paper, to gradually tweak their analysis in various ways, settling on methods that only seem like the best in retrospect. Most of the unfruitful avenues are not written down, or even remembered. The end result is the same.
Even if researchers are meticulously honest, their choices are often data-dependent. Suppose a researcher studying the impact of education on income finds an unexpectedly weak correlation in their data. This might cause them to wonder if outliers, such as exceptionally high earners, should be excluded, or if a particular subgroup, say recent immigrants, should be analyzed separately. Each choice depends heavily on the specific characteristics of the sample at hand. The statistician and political scientist Andrew Gelman likens this process to wandering through a garden of forking paths. If we had wandered down a different path, would we have produced approximately the same results?
One way to test for this kind of fragility in our published results is to have other researchers rerun the analyses using another justifiable approach. The Institute for Replication organizes large team efforts that do exactly this. Their work allows us to check whether results replicate at all and, if so, how robust the findings are to reasonable changes in the method of analysis. I participated in one of these for a paper on politically motivated reasoning. In my case, the results were quite robust. Do others hold up as well?
We have some systematic work examining this question, though it’s a bit hard to form a consistent overall picture. Brodeur’s analysis with I4R examined 110 papers recently published in top economic and political science journals. They found that about 70% of estimates that were statistically significant remained so after minor changes in methodology.
But another study published just a few years earlier paints a less optimistic picture. Seven economists were asked to re-analyze two research questions: 1) about the effects of compulsory schooling on teenage pregnancy and 2) about the impact of employer-based health insurance on entrepreneurship. The authors had the data from the original studies, but unlike the authors of the I4R paper, they were not working with the original analysis code. They had to wander the garden of forking paths alone, unable to rely on the choices made by previous researchers.
This time, the results were much messier. While all of the researchers made reasonable choices about data cleaning and analysis, nobody ended up with even the same sample size in their analysis. Statistical significance varied across studies, the magnitude of the effect swung widely, and for one study some of the replicators found a positive effect and some found a negative effect. This makes our work look very fragile indeed.
Let’s imagine — perhaps optimistically — that the 70% figure from the I4R study is more accurate. How worried should we be? Whether 70% robustness reproducibility is viewed as positive or negative depends a lot on your original expectations. But concretely, it seems fair to say that about 1 in 4 estimates, even from recent years, in our very best journals, do not stand up to modest changes in analytical approach.
Low power
Let us next consider problems that may appear in papers that clear our first two hurdles. We’re already reducing our sample dramatically, throwing out at least a quarter of results in the best journals (and many more in lower-ranked publications that lack open data and code). Can we rely on the results of these papers? Probably not, for reasons of statistical power.
To understand statistical power, it’s useful to recall why we have statistics in the first place: in large part, it’s to learn about a large population from a smaller sample. Statistics allows us to make good guesses about whole populations without measuring every member, and helps us quantify how good those guesses are.
Statistical power is the probability that you will find an effect in your sample when that effect actually exists in the population. Consider an exam designed to measure ’ math skills. Let’s say we want to compare two students — one who knows 60% of the material and another who knows 70%. Imagine our exam only has three questions. Because of the large role of luck, these two students will often end up with the same grade. Worse, when they do get different grades — say, one gets two questions right while the other gets three — the difference in scores appears larger than it actually is. With some random luck, the weaker student might even outperform the stronger one.
This three-question exam is a lot like an underpowered study. The effect of random noise (guessing, luck, or variability) is large relative to our ability to detect a real difference. As a result, we might wrongly conclude that the students are equally skilled, or that their skills differ by a huge amount.
We could fix this problem by replacing the three-question exam with a 50-question exam. Now, a single lucky guess or a brief lapse in focus won’t have as big an impact on the overall score. With more questions, the difference between students with different skill levels becomes clearer. A student who truly knows 60% of the material will more consistently score lower than a student who knows 70%, and that 10% difference will be easier to detect. The 50-question exam is more powerful.
A lot of studies in political science and economics are underpowered relative to the effect sizes they seek to detect. This means that when we find statistically significant results, they are often much larger than the true underlying effects. This happens in serious work by esteemed academics. For example, a randomized trial of an early-childhood intervention on Jamaican toddlers published in Science found a 25% increase in average adult earnings for the treatment group. The kids in the treatment group received only 2 years of help from community health workers, so this impact seems suspiciously large. But the study was small — just 105 children 4 — and the effect of this kind of treatment is likely to be quite variable. Like the low powered math exam, this study probably overestimated the true effect. This is one example, but this sort of problem affects most of the research published in economics and political science. We can see this across multiple lines of evidence, from studies based on meta-analysis to studies based on replications of experimental work.
For our policy maker, this means that even if the study they find clears the first two hurdles, its result will quite likely overestimate effects relative to reality. Crucially, if they were to plug this result into a cost-benefit analysis, it may be much too optimistic.
Causal research is expensive, time-consuming, and challenging. As a result, most of our research is underpowered to detect either the effect sizes that exist or the minimum effect sizes that policy makers care about. This means that, most of the time, we should be running studies and not finding statistically significant results. It may thus come as a bit of a surprise to learn that our journals are in fact filled with statistically significant results. This is both interesting and troubling, and it brings us to our next topic.
Selection on significance
Imagine you edit an academic journal. Part of your job is to decide which submitted articles to send for peer review and which to reject up front. In practice, a reasonable criterion would appear to be whether the article discovers something new. An idea or analysis might be new, but if the result cannot reject the null, it’s hard to make it attention grabbing. 5 In general, statistical significance is close to a necessary criterion for selection. As a result, your journal would increasingly be filled with such results.
This is, of course, how most journals run. But this creates an imbalance: after all, many experimental hypotheses will turn out to be false. Null results can be just as scientifically valuable as significant ones, but they’re a lot less likely to be published.
This is a problem for at least two reasons. First, it encourages authors to try different analyses on their sample until they get a statistically significant result. Historically, this sort of behavior was fairly common and (incorrectly) thought to be benign, but we’re well past that point now (at least in most disciplines). My own view is that this sort of fishing is essentially research malpractice. The problem here is somewhat akin to a game my kids play where they try to predict what’s for dinner by rapidly guessing different dishes. If we only count the last guess, their hit rate is 100%. The more analyses a researcher runs, the more likely they are to achieve a false positive result by chance. Still, it remains common practice for researchers to run many statistical tests — looking at different outcomes and different combinations of variables or in different subsets of the data — and then to report only the ones that worked. 6
The second problem is structural, and much deeper than some academics with questionable ethics. Even perfectly ethical researchers will produce published results that are often wrong if journals only accept significant findings. This is because all statistical tests involve some random error. By convention, we call a result “significant” if its p-value is below 0.05, meaning there’s less than a 5% probability of obtaining a result at least as large as the one a researcher found purely by chance, if we assume that no real effect exists. This means that even if there’s truly no effect, about 5% of studies will incorrectly appear significant. These are false positives. Because journals disproportionately publish significant findings, we end up publishing too many false positives and too few null results. This distorts our understanding of reality by making effects appear more common and robust than they truly are.
In other fields, this problem is well recognized. Many medical RCTs are pre-registered on ClinicalTrials.gov, which means researchers can compare the trials that have been run to the trials that have been published. A meta-science paper looking at antidepressant studies did just that. The researchers found, with surprisingly few exceptions, that trials with positive effects ended up published, whereas most studies that returned negative or null results were not published at all. The overall effect of this (unintentional) filter was that effect sizes in the published literature were inflated by nearly one-third.
This problem is worse in social science. The interaction between selection on significance and low power means that our published literature is often not merely kind of wrong but wildly wrong. Let’s go back to the math test example. Selecting for significance is like letting students take variations on the same exam many times and only reporting the top grade. If a 60% average student did this on a large (high powered) exam, then perhaps due to luck they’d end up with a 65%. A bias of 5 percentage points isn’t great, but it’s not devastating. However, if they did this on the low powered three-question exam then they’d very likely end up with 3/3 (100%) — a vast overestimation of their true ability.
The problem with our publishing system is not that underpowered studies like the early childhood intervention in Jamaica toddler sometimes find significant (and so, in expectation, inflated) effects. The problem is that we have informal rules that select only these studies for publication, leading to a systematically biased research record. If the Jamaican toddler study had found a tiny, statistically insignificant effect it would very likely have been rejected from Science (and perhaps not published at all). This is why meta-analyses of current research that do not try to correct for this publication bias produce results that are something like three times larger than results from pre-registered replications.
It isn’t everywhere, but it’s most places
All of this means that a policymaker might easily be worse off basing policy on peer-reviewed studies than on their own intuition. But this doesn’t mean we should give up on social science research altogether: there are many gems mixed in with the rocks. Meta-studies examining statistical power across economics and political science have found that while most research areas are full of underpowered research, around 10% have high statistical power relative to consensus effect sizes. This mirrors the kind of informal comments one will often get from academics, who will insist at the same time that most research is low quality while also excitedly telling you about a particular study they think is promising.
Concretely, most academics can point to findings in their fields that are replicable and important. For example, graduation programs — which combine modest asset transfers, training, and mentoring for very poor households — have been widely replicated and consistently shown to improve participants’ lives. I was part of an open evaluation of a meta-analysis on water treatment and child mortality, and I believe that the meta-analysis was important and well done. Teaching at the right level has received a lot of empirical support. The effects of deep canvassing are probably real. Important research on the drivers of support for democracy replicates.
It’s too early to be confident, but there’s some evidence that experts (at least in psychology) can probably identify which work will replicate. Prediction markets and machine learning approaches also look like they could be promising for identifying reliable research. One important future task is to figure out ways of surfacing this knowledge so people less immersed in the literature can take advantage of it.
Fixes
There are a lot of things that we can do to improve the quality of social science research. Many of these are cheap, some of them are being piloted, and some are being rolled out too slowly.
First, journals can mandate that authors provide public data and code when an article is published. A higher bar would be to ensure that the code actually runs, and that it is for the complete code pipeline — from raw data to results — whenever possible. Even better would be to make all this available to reviewers, to allow them to “kick the tires” on the analysis before deciding whether to accept the paper. Many journals do the first step, a few do the second, and while I am not aware of any that do the third, I expect that it probably happens somewhere.
Second, we can do more to actually check code, both to see if it runs and also to see if results are robust. Here, the best work is being done by the Institute for Replication (where I’m an editorial board member). Right now, AI seems to do a bad job at interpreting and running replication packages, but this may well change in the future. Perhaps some work around checking code can be at least partially automated. We should also recognize that most social scientists are neither programmers nor professional statisticians. One implication of this is that we should use well-tested packages when possible rather than implementing tricky methods in code ourselves. We’ll only get well-tested packages if we support their creation, and there we can do more to create an academic culture where software contributions rise in value.
Third, journals can do much more to fight selection on significance. The most promising idea in this space is the registered report, where studies are evaluated after they are designed but before data is collected or treatments are applied. There are numerous advantages to doing peer review at this point. A straightforward one is that reviewer comments can actually influence how the study is run. Registered reports also address publication bias because a paper will be reviewed and accepted for publication before any results are known. A few journals, like the Journal of Development Economics or American Political Science Review, currently accept registered reports, but they should be much more widely adopted.
Much observational statistical research isn’t a natural fit for registered reports. For this work, we need to experiment with ways to select articles for publication that do not merely select for statistical significance. Bayesian inference or frequentist equivalence testing are useful tools here, but what we really need is a culture shift around what it means for an article to be “interesting.” We’ll know that we are making progress when we see more precise null results testing plausible theories or programs and fewer statistically significant interaction effects or subgroup analyses (which are notorious for having lower power). In the medium term, if authors increase the statistical power of their tests (and reviewers and editors request such calculations), the bias created by filtering for statistical significance will be lessened, and maybe by a lot. Funders also can help by tying grants to error-catching or bias-reducing practices. Not to do so belies a desire for generating reliable evidence in the first place.
Our entire journal system was invented well before the internet and fails to take advantage of almost all of its potential. Today, we mainly use the internet to share digital versions of printed pages, making it little more than a faster postal service. This is a shame because the internet gives us the potential to have living literature reviews, articles with accompanying code, and comments and discussion sections alongside articles.
Going further, AI gives us the ability to read literatures at vast scale and extract and categorize the resulting information. One can imagine publishing approaches that lean into this by making more aspects of research machine readable, allowing better synthesis at scale. (Of course, this may run counter to the commercial interests of publishers, so improvements in science may be blocked by paywalls.)
One promising idea around the evaluation of research is open evaluation, ideally with data and code. This, for example, is done at The Unjournal. From the first public evaluation, readers can see which aspects of the research evaluators found credible, what doubts they had, and how they think it should be used. Suppose this became a repeated process, as research projects were evaluated and improved. Policymakers could see how many times a study was evaluated, how detailed and careful these evaluations were, and the general consensus of the various reviewers. More experiments along these lines would be very welcome.
Regardless of how radical one wants to be with change, the current system for producing research is broken. We are wasting time, money, and skill.
The good news is that we already possess many of the tools to produce higher-quality social science. What’s missing is not mere “will,” but better incentives and coordination. Journals, funders, and academic departments all need to shift their reward structures so that transparency, replication, and rigor are prized at least as much as novelty. That kind of coordinated change can be slow and messy, but it’s feasible. A few pioneering journals are already experimenting with registered reports, and many journals now have improved replication policies. Policy-relevant research stands to benefit enormously when these sorts of changes become widespread. If the gatekeepers of academic prestige and resources move first, a healthy new norm could take root — and ultimately make our research worthy of real-world decision-making.
- I will focus on economics and political science because they are doing the best on the research practices that I flag as problematic. Fields like sociology or criminology are noticeably behind. ↩
- These were all published in 2016. They sampled 415 articles and were left with 203 were empirical papers after dropping those that contained proprietary or restricted data. They gave themselves four hours per paper to try to get the code to run before deeming it not reproducible. ↩
- These sort of coding errors were about 60% more common in economics than political science, perhaps because the former tends to have more lines of code. ↩
- Out of 129 originally included. (The follow-up was done over 20 years later.) ↩
- Part of getting more null results published depends on pitching them better, using things like equivalence tests to show effect sizes that one can rule out with some level of confidence. ↩
- You might think the tendency in economics or political science to demand very many robustness tests would fully address this concern, but that is a misunderstanding of the problem. A major problem with p-hacking is the hypothesis was selected for significance, which can happen due to unlucky sampling. In that situation, trying various specifications will not help. ↩